6.4 Association Rules
The ore.odmAssocRules
function implements the Apriori algorithm to find frequent itemsets and generate an association model.
The function finds the co-occurrence of items in large volumes of transactional data such as in market basket analysis. An association rule identifies a pattern in the data in which the appearance of a set of items in a transactional record implies another set of items. The groups of items used to form rules must pass a minimum threshold according to how frequently they occur (the support of the rule) and how often the consequent follows the antecedent (the confidence of the rule). Association models generate all rules that have support and confidence greater than user-specified thresholds. The Apriori algorithm is efficient, and scales well with respect to the number of transactions, number of items, and number of itemsets and rules produced.
The formula
specification has the form ~ terms
, where terms
is a series of column names to include in the analysis. Multiple column names are specified using +
between column names. Use ~ .
if all columns in the data should be used for model building. To exclude columns, use -
before each column name to exclude. Functions can be applied to the items in terms
to realize transformations.
The ore.odmAssocRules
function accepts data in the following forms:
-
Transactional data
-
Multi-record case data using item id and item value
-
Relational data
For examples of specifying the forms of data and for information on the arguments of the function, call help(ore.odmAssocRules)
.
The function rules
returns an object of class ore.rules
, which specifies a set of association rules. You can pull an ore.rules
object into memory in a local R session by using ore.pull
. The local in-memory object is of class rules
defined in the arules
package. See help(ore.rules)
.
The function itemsets
returns an object of class ore.itemsets
, which specifies a set of itemsets. You can pull an ore.itemsets
object into memory in a local R session by using ore.pull
. The local in-memory object is of class itemsets
defined in the arules
package. See help(ore.itemsets)
.
Settings for an Association Rules Model
The following table lists the settings that apply to Association Rules models.
Table 6-4 Association Rules Model Settings
Setting Name | Setting Value | Description |
---|---|---|
ASSO_ABS_ERROR |
|
Specifies the absolute error for the association rules sampling. A smaller value of The default value is |
|
A comma separated list of strings containing the names of the columns for aggregation. Where the number of columns in the list must be |
Specifies the columns to aggregate. You can set An item value is not mandatory. The default value is For each item, you may supply several columns to aggregate. However, doing so requires more memory to buffer the extra data and also affects performance because of the larger input data set and increased operations. |
|
A comma separated list of strings, at least one of which must appear in the antecedent part of each reported association rule. |
Sets Including Rules for the antecedent. The default value is |
|
A comma separated string containing the list of excluded items that none of them can appear in the consequent part of each reported association rule. |
Sets Excluding Rules for the consequent. You can use the excluding rule to reduce the data that must be stored, but you might need to build extra models to run different Including or Excluding Rules. The default value is |
|
|
Specifies the confidence level for an association rules sample. A larger value of |
|
A comma separated list of strings, at least one of which must appear in the consequent part of each reported association rule. |
Sets Including Rules for the consequent. The default value is |
|
A comma separated list of strings, none of which can appear in the consequent part of a reported association rule. |
Sets Excluding Rules for the consequent. You can use the Excluding Rule to reduce the data that must be stored, but you may be required to build extra models for executing different Including or Excluding Rules. The default value is |
ASSO_EX_RULES |
A comma separated list of strings that cannot appear in an association rule. |
Sets Excluding Rules applied for each association rule. No rule can contain any item in the list. The default value is |
|
A comma separated list of strings, at least one of which must appear in each reported association rule, either as antecedent or as consequent |
Sets Including Rules applied for each association rule. The default value |
ASSO_MAX_RULE_LENGTH |
TO_CHAR(2 <= X <= 20) |
Maximum rule length for association rules. The default value is |
ASSO_MIN_CONFIDENCE |
TO_CHAR(0 <= X <= 1) |
Minimum confidence for association rules. The default value is |
ASSO_MIN_REV_CONFIDENCE |
TO_CHAR(0 <= X <= 1) |
Sets the Minimum Reverse Confidence that each rule should satisfy. The Reverse Confidence of a rule is defined as the number of transactions in which the rule occurs divided by the number of transactions in which the consequent occurs. The value is real number between 0 and 1. The default value is |
|
TO_CHAR(0 <= X <= 1) |
Minimum support for association rules. The default value is |
|
TO_CHAR(0 <= X <= 1) |
Minimum absolute support that each rule must satisfy. The value must be an integer. The default value is |
|
column_name |
The name of a column that contains the items in a transaction. When you specify this setting, the algorithm expects the data to be presented in native transactional format, consisting of two columns:
|
ODMS_ITEM_VALUE_COLUMN_ NAME |
column_name |
The name of a column that contains a value associated with each item in a transaction. Use this setting only when you have specified a value for If you also use
If The Item Value column may specify information such as the number of items (for example, three apples) or the type of the item (for example, macintosh apples). |
Example 6-3 Using the ore.odmAssocRules Function
This example builds an association model on a transactional data set. The packages arules
and arulesViz
are required to pull the resulting rules and itemsets into the client R session memory and be visualized. The graph of the rules appears in the figure following the example.
# Load the arules and arulesViz packages. library(arules) library(arulesViz) # Create some transactional data. id <- c(1, 1, 1, 2, 2, 2, 2, 3, 3, 3, 3) item <- c("b", "d", "e", "a", "b", "c", "e", "b", "c", "d", "e") # Push the data to the database as an ore.frame object. transdata_of <- ore.push(data.frame(ID = id, ITEM = item)) # Build a model with specifications. ar.mod1 <- ore.odmAssocRules(~., transdata_of, case.id.column = "ID", item.id.column = "ITEM", min.support = 0.6, min.confidence = 0.6, max.rule.length = 3) # Generate itemsets and rules of the model. itemsets <- itemsets(ar.mod1) rules <- rules(ar.mod1) # Convert the rules to the rules object in arules package. rules.arules <- ore.pull(rules) inspect(rules.arules) # Convert itemsets to the itemsets object in arules package. itemsets.arules <- ore.pull(itemsets) inspect(itemsets.arules) # Plot the rules graph. plot(rules.arules, method = "graph", interactive = TRUE)
Listing for This Example
R> # Load the arules and arulesViz packages.
R> library(arules)
R> library(arulesViz)
R> # Create some transactional data.
R> id <- c(1, 1, 1, 2, 2, 2, 2, 3, 3, 3, 3)
R> item <- c("b", "d", "e", "a", "b", "c", "e", "b", "c", "d", "e")
R> # Push the data to the database as an ore.frame object.
R> transdata_of <- ore.push(data.frame(ID = id, ITEM = item))
R> # Build a model with specifications.
R> ar.mod1 <- ore.odmAssocRules(~., transdata_of, case.id.column = "ID",
+ item.id.column = "ITEM", min.support = 0.6, min.confidence = 0.6,
+ max.rule.length = 3)
R> # Generate itemsets and rules of the model.
R> itemsets <- itemsets(ar.mod1)
R> rules <- rules(ar.mod1)
R> # Convert the rules to the rules object in arules package.
R> rules.arules <- ore.pull(rules)
R> inspect(rules.arules)
lhs rhs support confidence lift
1 {b} => {e} 1.0000000 1.0000000 1
2 {e} => {b} 1.0000000 1.0000000 1
3 {c} => {e} 0.6666667 1.0000000 1
4 {d,
e} => {b} 0.6666667 1.0000000 1
5 {c,
e} => {b} 0.6666667 1.0000000 1
6 {b,
d} => {e} 0.6666667 1.0000000 1
7 {b,
c} => {e} 0.6666667 1.0000000 1
8 {d} => {b} 0.6666667 1.0000000 1
9 {d} => {e} 0.6666667 1.0000000 1
10 {c} => {b} 0.6666667 1.0000000 1
11 {b} => {d} 0.6666667 0.6666667 1
12 {b} => {c} 0.6666667 0.6666667 1
13 {e} => {d} 0.6666667 0.6666667 1
14 {e} => {c} 0.6666667 0.6666667 1
15 {b,
e} => {d} 0.6666667 0.6666667 1
16 {b,
e} => {c} 0.6666667 0.6666667 1
R> # Convert itemsets to the itemsets object in arules package.
R> itemsets.arules <- ore.pull(itemsets)
R> inspect(itemsets.arules)
items support
1 {b} 1.0000000
2 {e} 1.0000000
3 {b,
e} 1.0000000
4 {c} 0.6666667
5 {d} 0.6666667
6 {b,
c} 0.6666667
7 {b,
d} 0.6666667
8 {c,
e} 0.6666667
9 {d,
e} 0.6666667
10 {b,
c,
e} 0.6666667
11 {b,
d,
e} 0.6666667
R> # Plot the rules graph.
R> plot(rules.arules, method = "graph", interactive = TRUE)
Figure 6-1 A Visual Demonstration of the Association Rules
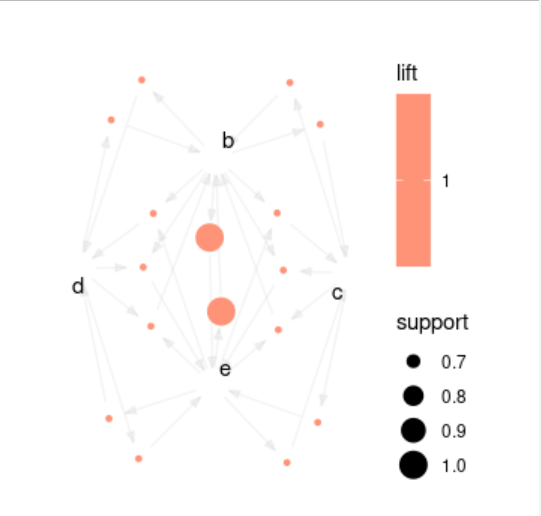
Description of "Figure 6-1 A Visual Demonstration of the Association Rules"